The Impact of Data Quality on Research Reports: Why Accurate Data Matters
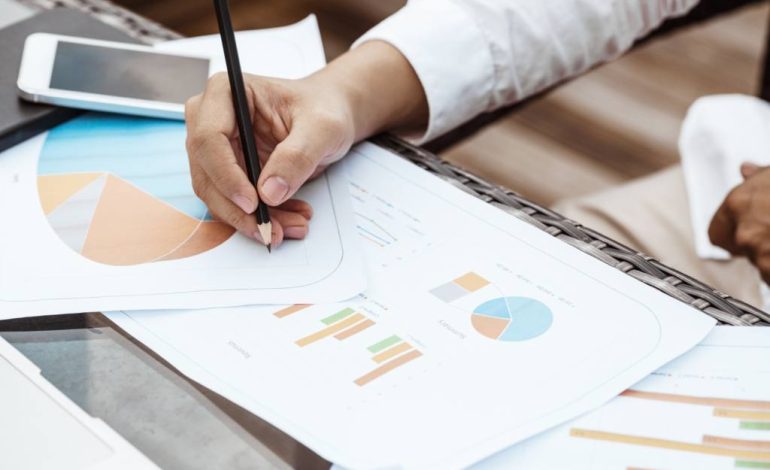
In today’s data-driven world, the quality of data stands as a pillar of success for analysts, data scientists, and business professionals. Accurate data forms the foundation for insightful research reports and sound decision-making. Without it, even the most sophisticated analyses can lead to misleading conclusions, affecting businesses and scientific communities alike.
This blog post aims to shed light on the profound impact of data quality on research reports and business decisions. We will explore why accurate data matters, how to measure it, and the best practices to ensure high data quality. By the end, you’ll understand why prioritizing data quality is indispensable for achieving reliable and impactful outcomes.
The Significance of Accurate Data
Accurate data serves as the lifeblood of any research report. When data is accurate, it ensures that insights derived from it are precise and trustworthy. Analysts and data scientists rely heavily on this accuracy to make informed recommendations and predictions.
The risks of using poor quality data are immense. It can lead to incorrect conclusions, misguided business strategies, and a loss of credibility. For instance, if a company bases its market expansion strategy on flawed data, it may enter a region with low demand, resulting in significant financial losses. Thus, accurate data is crucial for minimizing risks and enhancing the reliability of research.
Furthermore, accurate data builds trust. Stakeholders are more likely to support decisions and strategies backed by precise data. This trust is essential for maintaining strong relationships with clients, investors, and other key players.
Data Quality Metrics
Assessing data quality involves evaluating several key metrics. These metrics help ensure that the data used in research is fit for its intended purpose. Here are the four primary metrics:
Accuracy
Accuracy refers to the correctness of data. Accurate data correctly represents the real-world values it is supposed to depict. For example, an accurate customer database will correctly list each customer’s name and contact details.
Completeness
Completeness measures whether all required data is present. Incomplete data can lead to gaps in analysis and faulty conclusions. For example, if half of the entries in a sales report are missing, the report will not provide a true picture of the company’s performance.
Consistency
Consistency ensures that data is uniform across different datasets and systems. Inconsistent data can cause confusion and errors in analysis. For example, if one system lists a product price as $100 and another as $105, this inconsistency can disrupt financial analysis.
Timeliness
Timeliness assesses whether data is up-to-date and available when needed. Outdated data can mislead analysts and result in decisions based on obsolete information. For example, using last year’s market trends to predict next year’s sales could lead to inaccurate forecasts.
Impact on Research and Reporting
The quality of data directly influences the outcomes of research and the credibility of reports. High-quality data leads to accurate insights, while poor-quality data can distort findings and damage the credibility of the research.
Case Study: Healthcare Research
In healthcare research, accurate data is critical. A study relying on patient data to determine the effectiveness of a new drug must ensure the data is precise and current. If the data is flawed, the study’s conclusions could be incorrect, potentially leading to the approval of an ineffective or harmful medication.
Business Reporting
For businesses, data quality impacts financial reporting and strategic planning. A company using inaccurate sales data may overestimate its revenue, leading to poor investment decisions and financial instability. Accurate data, on the other hand, enables businesses to make informed decisions, optimize operations, and maintain financial health.
Best Practices for Ensuring Data Quality
Ensuring high data quality requires a proactive approach. Here are some practical tips to help improve data quality within your organization:
Data Cleaning
Data cleaning involves correcting or removing inaccurate, incomplete, or irrelevant data. This process helps ensure that the data used in analysis is accurate and reliable. Techniques include removing duplicates, correcting errors, and standardizing formats.
Validation Processes
Implementing validation processes helps verify data accuracy before it is used in analysis. This can include automated checks for data entry errors, consistency checks across systems, and manual reviews by data experts.
Use of Reliable Sources
Using data from reliable sources is essential for maintaining data quality. Trusted sources are more likely to provide accurate and up-to-date information. For example, government databases and reputable industry reports are generally reliable sources of data.
The Future of Data Quality in Research
The landscape of data quality is continuously evolving, with emerging trends and challenges shaping its future. Here are some insights on what to expect:
Advanced Data Quality Tools
New tools leveraging AI and machine learning are emerging to enhance data quality. These tools can automatically detect and correct errors, predict data issues, and provide recommendations for improvement.
Increasing Volume and Variety of Data
With the rise of big data, organizations are dealing with larger volumes and more diverse types of data. Ensuring the quality of such data presents new challenges, requiring more sophisticated techniques and tools.
Continuous Improvement
The importance of continuous improvement in data quality cannot be overstated. Organizations must regularly review and update their data quality practices to keep pace with changing data landscapes and technological advancements.
Conclusion
In summary, the quality of data is fundamental to the success of research reports and business decisions. Accurate data drives precision, builds trust, and minimizes risks. By understanding data quality metrics and implementing best practices, organizations can enhance the reliability and impact of their research.
Prioritizing data quality is not just a best practice; it is a necessity in today’s data-driven world. Start by evaluating your current data quality practices and seek ways to improve them. Remember, high-quality data is the key to unlocking reliable insights and achieving business success.